Harness the power of AI to optimize your newly commissioned data centers to be more reliable and sustainable.
In today’s dynamic landscape of data center operations, the stakes are higher than ever. With customers demanding greater sustainability, governments enforcing stringent energy regulations, and extreme weather events on the rise, data center operations are getting exponentially more complex.
How can you maintain PUE and WUE targets in data centers while ensuring uptime?
AI simplifies operations to improve sustainability and reliability. Using existing BMS and power sensor data, you’ll eliminate the costs and time required for complex IoT projects. Driving a short time-to-value of mere weeks.
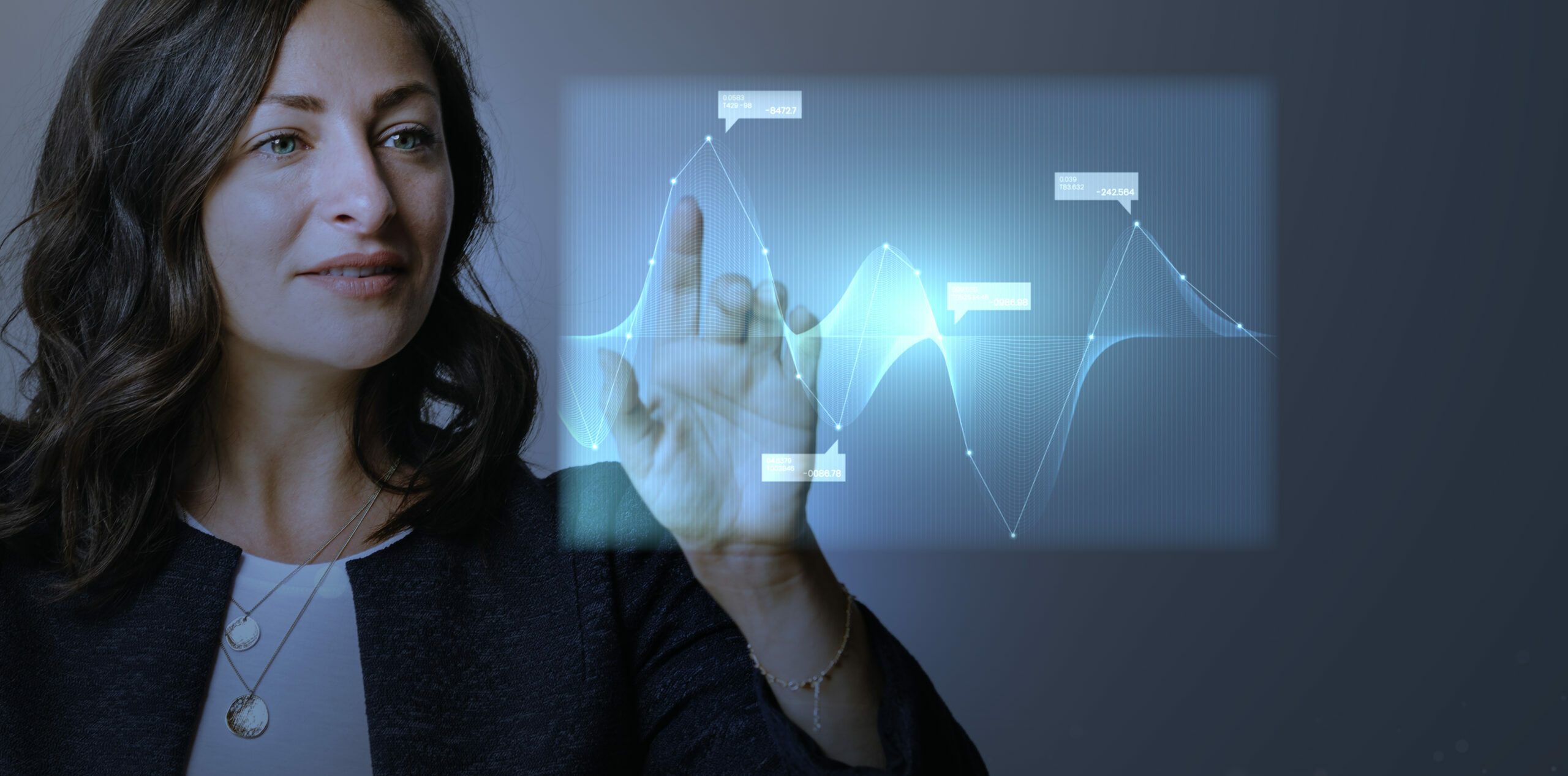
Optimizing a new data center
Our track record speaks for itself. We have optimized new and legacy data centers globally, resulting in over 18,500MWh of proven energy savings for our customers (read our prior update about how achieved the 14,000MWh milestone).
One recent success story involved a 20MW state-of-the-art data center equipped with modern hybrid cooling systems. In a few short months:
- We achieved over 4,000MWh per year in energy savings,
- Met PUE targets, and
- Enhanced asset reliability
- All while empowering the operations team to focus on mission-critical tasks
We quickly identified and quantified considerable optimizations after integrating the sensor data into our Coolgradient AI platform. Most notably:
- Energy losses from the hybrid cooling units operating in mechanical mode when outside air temperatures were as low as 8C/46F.
- Potential operational risk increase since many cooling units were operating at the same time.
Getting to the root cause
Being able to process hundreds of billions of sensor readings with AI, enables two things:
- Automated and advanced analysis of complex patterns, as happens continuously within any data center.
- Pinpointing where the causes are, despite the sheer number of possibilities, and ensuring effective mitigating measures.
The main causes for the huge optimizations for this particular data center revolved around three areas. First, hysteresis in the free cooling control strategy had a cascading effect, resulting in mechanical mode operating in the winter period.
Second, our ML (Machine Learning) models identified high margins between the pumps’ lower and upper sequencing setpoints and high differential pressure in the chilled water ring. This resulted in too many hybrid cooling units running simultaneously at high speeds, resulting in high energy consumption.
Furthermore, our data center optimization software also identified that the Adiabatic cooling was only operational in some chillers and not in others. When the Adiabatic mode was off, it resulted in higher energy consumption. Another opportunity to reduce energy consumption.
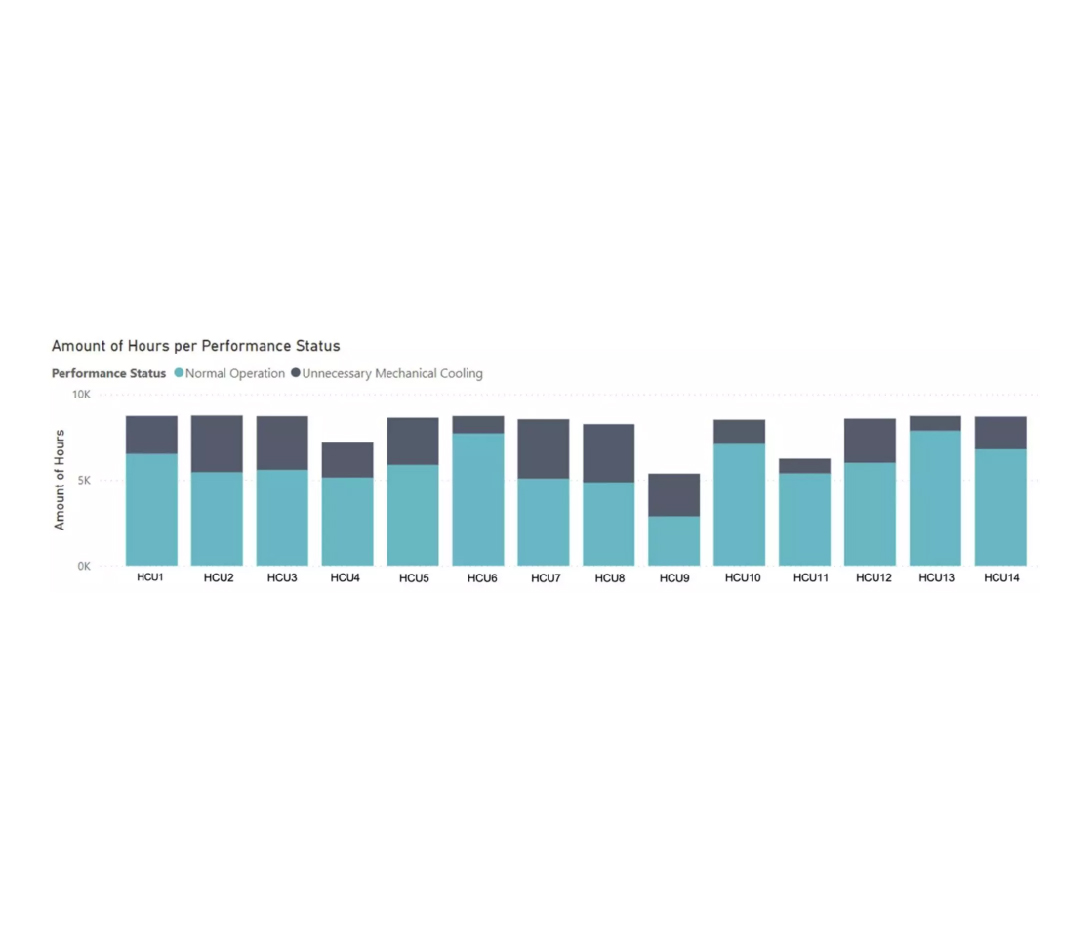
For example, the graph above shows the free cooling operation per Hybrid Cooling Unit (HCU) for a given period. The graph also shows the missed opportunity to run in free cooling mode, marked as ‘unnecessary mechanical cooling.’ Analysis and visualizations like these are automatically part of any implementation of our platform – depending on the data center infrastructure, of course – and drive swift optimizations in a matter of weeks. In this case, the amount of ‘unnecessary mechanical cooling’ hours totaled thousands yearly.
Effective measures and evaluating its impact
Having this level of detail available from your whole data center allows for effectively determining and implementing mitigating measures.
In this case, and benchmarking performance data from other data centers, our specialist team recommended adopting the control strategy and advised the local operations team to reduce the differential pressure step by step, quantifying the benefits. Next, they introduced a plan to increase the sequencing setpoint, which resulted in fewer hybrid cooling units running simultaneously.
Using already available advanced monitoring platform capabilities, the impact of the (setting) changes was tracked to ensure the entire cooling ecosystem ran harmoniously as soon as possible.
Next steps
The customer is currently onboarding the next 20MW data center on the same campus. Using our AI platform and learnings from the neighboring data center, they can deliver smooth customer onboarding while achieving PUE, WUE, and customer SLAs.
This is one example of many on our mission to make data centers more intelligent and empower teams to do their best work.
Having our AI optimization platform by their side simplifies the complex operation environment for any local operations team. This allows them to stay on top of relevant optimization opportunities and focus their scarce time on the most impactful items. With the support of our advisory services teams, the client operations teams and energy champions continuously discuss best practices on changes to improve sustainability and decrease uptime risks.
Are your data centers operating at their full potential? Do not let inefficiencies go unnoticed. Contact Coolgradient today to learn how our technology and expert team can help you deliver improved reliability and reduced energy costs.
Stay cool, stay optimized, with Coolgradient.